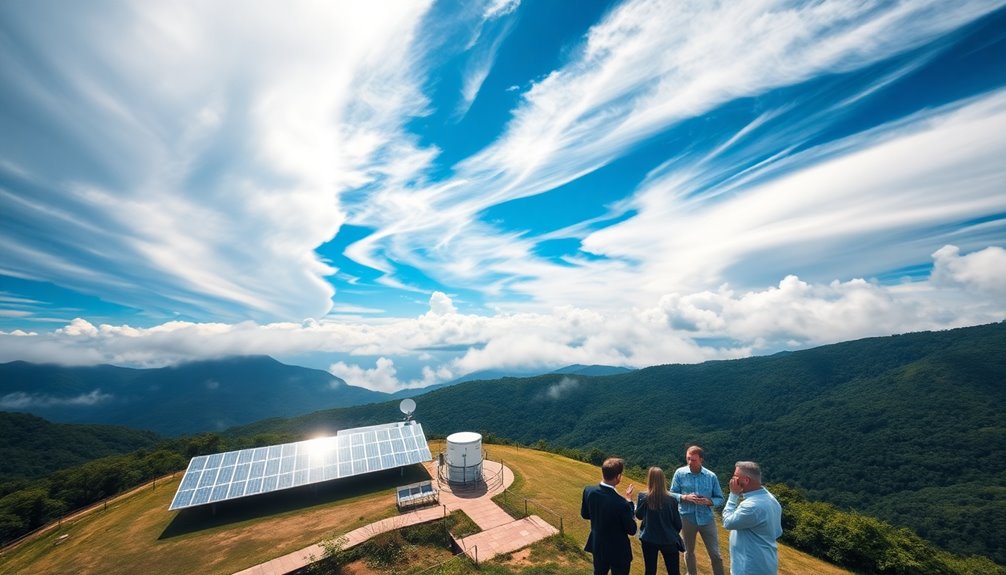
DeepMind Revolutionizes Weather Forecasting Tech
December 5, 2024DeepMind's introduction of the GenCast AI model marks a significant advancement in weather forecasting, achieving remarkable efficiency and accuracy improvements over traditional methods. With the capability to produce detailed 15-day forecasts in just eight minutes and a notable enhancement in predicting wind power output, this technology raises important questions about its implications for the meteorological community. As the potential for collaboration and innovation grows, it is essential to evaluate the challenges that may accompany this shift in forecasting paradigms. What does this mean for the future of climate prediction?
AI vs. Traditional Forecasting Methods
As traditional weather forecasting methods continue to face the growing demands of accuracy and computational efficiency, artificial intelligence (AI) has emerged as a viable alternative that can keep pace with, and in some cases surpass, these conventional approaches.
Traditional accuracy in weather predictions often relies on complex atmospheric models, which, while highly effective, can be computationally intensive and slow. In contrast, AI-driven models like DeepMind's GenCast demonstrate competitive performance, although they also face AI limitations, particularly in maintaining specificity over extended forecasting periods.
While AI shows promise in enhancing forecasting capabilities, its effectiveness is frequently contingent on the quality of training data, particularly in regions with sparse observational networks, raising questions about the overall reliability compared to traditional methods.
Computational Efficiency Gains
Significant advancements in computational efficiency have been achieved with the introduction of DeepMind's GenCast model, which can generate a detailed 15-day weather forecast in just eight minutes on Google's high-performance processors.
This remarkable GenCast optimization allows for the execution of multiple instances in parallel, enabling ensemble simulations to be completed in under 20 minutes.
The model's low computational requirements not only enhance accessibility for academic research teams but also encourage broader experimentation with ensemble forecasting techniques.
By utilizing diffusion models, GenCast effectively evolves noise patterns into realistic weather data, providing higher resolution forecasts compared to traditional methods.
These efficiencies position GenCast as a transformative tool for weather forecasting, pushing the boundaries of what is possible in climate prediction.
Performance Metrics and Success
In evaluating the performance of DeepMind's GenCast, the model has demonstrated remarkable success across various metrics, consistently outperforming traditional forecasting systems.
Through rigorous benchmark tests, GenCast has achieved superior results, particularly in extreme weather scenarios and tropical cyclone tracking.
- Outperformed traditional models in 97% of benchmark tests across future timeframes.
- Achieved a 20% accuracy improvement in wind power output forecasts for initial days.
- Maintained high accuracy during early forecast periods while addressing rare weather events.
These performance evaluations showcase GenCast's potential to redefine weather forecasting, offering a blend of accuracy and computational efficiency that traditional models struggle to match.
The ongoing research will further enhance its capabilities, ensuring sustained improvements in predictive reliability.
Challenges Facing AI Models
The effectiveness of AI models like DeepMind's GenCast is closely linked to the quality of training data, which presents a fundamental challenge in various regions.
In areas with dense observational networks, AI can achieve remarkable accuracy; however, the situation is markedly different in regions with sparse data.
Data accessibility is a critical issue, particularly in lower-income countries where financial constraints restrict meteorological departments from sharing essential information. This limitation hampers the potential of AI models, as they depend heavily on thorough datasets for effective training.
Consequently, the reliance on reanalysis data becomes essential, yet it may not fully address the gaps in real-time observational data, thereby impacting the overall performance of AI-driven weather forecasting systems.
Future of Weather Forecasting
Advancements in AI-driven models like DeepMind's GenCast highlight a promising direction for the future of weather forecasting. The integration of hybrid approaches that combine AI with traditional meteorological methods is expected to enhance predictive accuracy and efficiency.
Key aspects of this evolution include:
- Improved handling of extreme weather events through advanced AI techniques.
- Increased data accessibility fostering collaboration and innovation among researchers worldwide.
- Streamlined computational processes that enable rapid forecasting, making timely decisions possible for various sectors.
As the field progresses, ongoing research will focus on refining these models and ensuring that training data remains robust and thorough, ultimately benefiting global climate forecasting efforts.
The future of weather forecasting looks brighter with these innovative approaches.