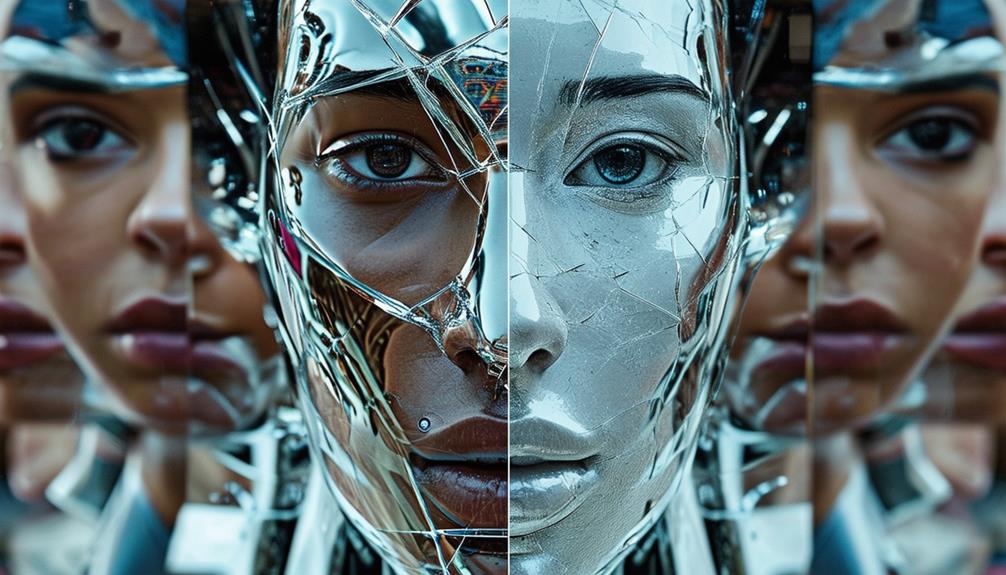
Understanding Ethical AI: Biased Data's Impact on Ethics
June 10, 2024You've likely encountered AI systems that make unfair decisions, perpetuating biases and prejudices. This is because biased data is a pervasive problem in AI development. Historical prejudices, inadequate data preprocessing, and lack of diversity in data collection all contribute to biased AI systems. These biases lead to unfair outcomes, discriminatory practices, and a loss of trust in AI. To build fair and ethical AI systems, you need to understand the sources of bias, mitigate bias in decision-making, and prioritize transparency and fairness. As you explore this complex issue, you'll discover the importance of addressing biased data to guarantee responsible AI development.
The Bias Problem in AI Development
As you develop AI systems, you're likely unaware that the data used to train machine learning models is often tainted with historical prejudices, perpetuating biases that can have far-reaching consequences.
These biases can lead to unfair outcomes, discriminatory practices, and unethical decision-making. The quality of your AI algorithms heavily depends on the diversity and representativeness of the training data. If your data is biased, your AI system will be too.
To address this, prioritizing transparency and continuous monitoring to detect and mitigate biases over time is critical. Diverse development teams can also help reduce the introduction of unconscious biases into AI algorithms.
Adhering to ethical guidelines and standards is vital for responsible AI development and addressing bias issues. By being aware of these potential pitfalls, you can take steps to promote fairness and transparency in your AI systems. This is fundamental for developing responsible AI that aligns with ethical concerns and promotes fairness in decision-making.
Understanding AI Ethics and Fairness
You're likely familiar with the concept of ethics in human decision-making, but when it comes to AI, ethics and fairness take on a whole new dimension. AI ethics involves promoting transparency, accountability, and responsibility in algorithmic decision-making. Fairness in AI, on the other hand, entails eliminating biases, avoiding discrimination, and considering training data and evaluation metrics.
To achieve fairness in AI, it's vital to:
- Eliminate biased data that can lead to unjust outcomes
- Implement fairness-aware algorithms that prevent discriminatory practices
- Foster collaborative efforts to address bias and maintain fairness
- Align AI practices with societal values to guarantee equal treatment
Without ethical AI practices, biased data can perpetuate societal biases in AI algorithms, leading to discriminatory practices. It's important to understand AI ethics and fairness to develop algorithms that align with societal values and prevent unjust outcomes.
Sources of Bias in Machine Learning
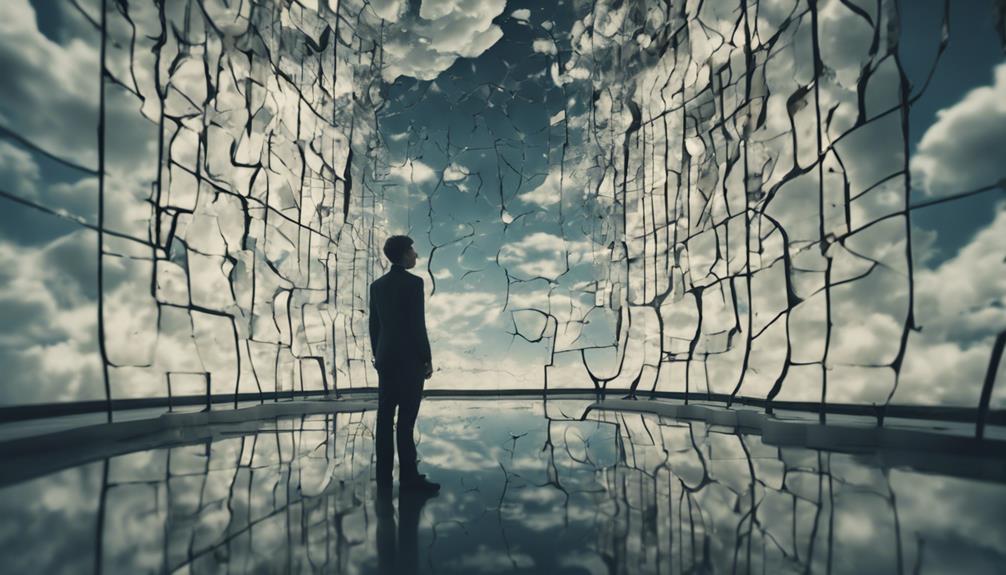
Identify the sources of bias in machine learning, and you'll likely uncover a complex web of influences that can compromise the fairness and transparency of your AI systems. When it comes to machine learning, bias can originate from various sources, including skewed training data, human prejudices, and algorithmic design flaws.
Historical biases present in training datasets can perpetuate societal prejudices and inequalities in machine learning models, raising ethical concerns regarding fairness in machine learning. Additionally, inadequate data preprocessing, unrepresentative samples, and biased labeling can contribute to the propagation of bias in machine learning.
Lack of diversity in data collection, biased data sources, and unbalanced feature representations can also introduce bias into machine learning algorithms. Moreover, external factors, lack of bias identification processes, and competitive pressures can influence the development of biased machine learning models, leading to unintended biases and algorithmic bias that reflect gender or racial prejudices.
To promote ethical principles are upheld, it's vital to recognize and address these sources of bias, mitigating their impact on AI systems and promoting fairness and transparency.
The Impact of Biased Data on AI
When you feed biased data into AI systems, you risk perpetuating societal prejudices and inequalities, which can lead to unfair outcomes in decision-making processes. This is because AI models trained on biased data may learn to replicate and amplify these biases, resulting in discriminatory practices in areas like hiring, lending, and criminal justice.
The impact of biased data on AI is far-reaching, with significant ethical implications. Here are just a few examples:
- Discriminatory outcomes: Biased data can lead to unfair treatment of certain groups, perpetuating existing social inequalities.
- Lack of fairness: AI systems trained on biased data may not make decisions based on merit, but rather on preconceived notions and stereotypes.
- Unethical decision-making: Biased data can result in AI algorithms making decisions that are unfair, unjust, and even harmful.
- Erosion of trust: When AI systems perpetuate biases, they can erode trust in institutions and undermine the legitimacy of decision-making processes.
To build ethical AI systems that prioritize fairness and inclusivity, it's essential to address biased data and ensure that AI algorithms are trained on high-quality, unbiased training data.
Mitigating Bias in AI Decision-Making
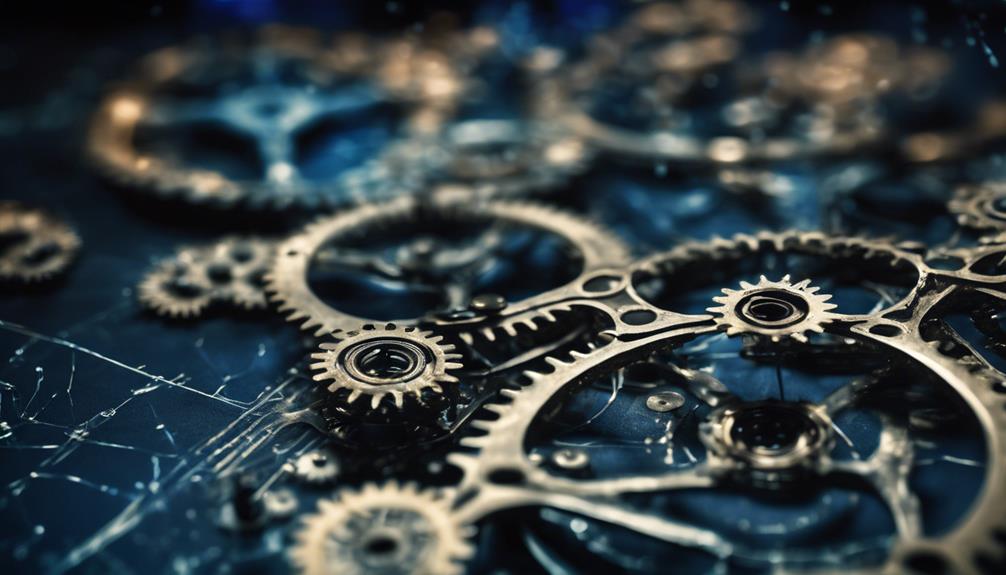
As you work to mitigate bias in AI decision-making, you'll need to focus on data preprocessing strategies that can help identify and correct biased data.
Additionally, you'll want to implement algorithmic bias detection techniques that can pinpoint and address biases in your AI systems.
Data Preprocessing Strategies
You can significantly reduce bias in AI decision-making by employing strategic data preprocessing techniques. This includes data cleaning, normalization, and feature engineering to create a more balanced and fair dataset. This is vital in mitigating bias in AI decision-making, as poor quality data can lead to unfair outcomes.
To guarantee fair AI models, you should:
- Remove outliers and handle missing values to prevent skewed results
- Balance class distributions using techniques like oversampling, undersampling, and synthetic data generation
- Apply dimensionality reduction methods to reduce features and improve model performance
- Select relevant features using feature engineering to prevent biased models.
Algorithmic Bias Detection
By implementing strategic data preprocessing strategies, you've taken the first step in reducing bias in AI decision-making, but now it's time to turn your attention to detecting and mitigating bias in the algorithms themselves. This is where algorithmic bias detection comes in – a critical step in ensuring ethical AI decision-making.
To detect bias, you'll need to conduct bias audits and analyze your AI model's outcomes using fairness metrics like disparate impact analysis and equalized odds. These metrics help identify biases in your AI system, which can then be addressed through bias mitigation strategies.
It's vital to analyze your training data, evaluate your model's outcomes, and implement bias mitigation techniques to minimize algorithmic bias. By doing so, you'll be able to develop artificial intelligence and machine learning models that make fair and ethical decisions.
The Role of Transparency in AI Ethics
As you explore the role of transparency in AI ethics, you'll find that explainable AI models are essential in providing insight into algorithmic decision-making.
By making decision paths visible, you can identify potential biases and guarantee that AI systems are fair and trustworthy.
Explainable AI Models
Delving into the complexities of AI decision-making, explainable AI models provide an essential layer of transparency, allowing you to peek under the hood and grasp how predictions are generated. This transparency is important for ensuring ethical AI practices.
With explainable AI, you can:
- Identify biases: Understand how AI algorithms make decisions and pinpoint biases that may lead to unfair outcomes.
- Promote accountability: Make AI systems accountable by providing a clear understanding of their decision-making processes.
- Verify fairness: Guarantee AI decisions are fair and unbiased by examining the reasoning behind predictions.
- Mitigate bias: Take corrective action to eliminate biases and ensure ethical implications are considered.
Visible Decision Paths
Transparency in AI decision paths is essential for you to uncover the inner workings of algorithms, ensuring that accountability and trust are built into every prediction.
By making decision paths visible, you can identify potential biases and ethical concerns in machine learning models. This transparency allows you to scrutinize and evaluate the decision-making process, ensuring it aligns with ethical guidelines.
Visible decision paths help prevent biases from being hidden or perpetuated, promoting fairness and ethical practices in AI development.
When you understand the decision paths of AI algorithms, you can identify and mitigate biases, leading to more responsible AI development.
Transparent AI models enable stakeholders to assess fairness and ethical considerations, promoting accountability in AI ethics.
Building Fairness Into AI Systems
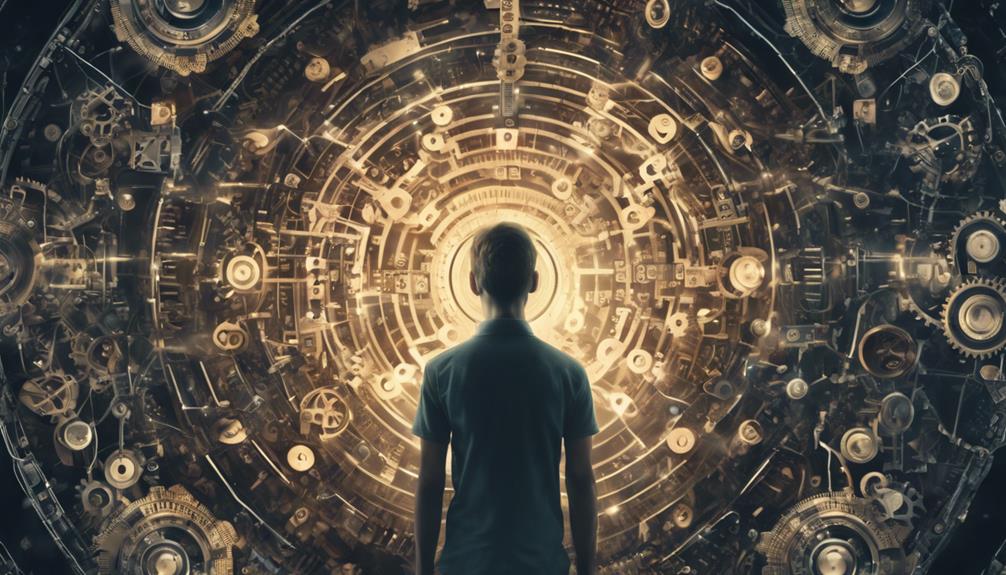
To build fairness into AI systems, you must consider the far-reaching implications of biased data on algorithmic decision-making. Biased data can lead to unfair outcomes, perpetuating existing social inequalities. It's important to address these biases in data analytics to construct fair and ethical AI systems.
To guarantee fairness, you should:
- Foster transparent and diverse development teams to mitigate the introduction of unconscious biases in AI.
- Establish ethical guidelines and standards to guide responsible AI development with fairness considerations.
- Prioritize human-centered design to address ethical concerns and promote fairness in algorithmic decision-making.
- Use unbiased data to train AI models, ensuring equitable outcomes and responsible AI development.
Ensuring Responsible AI Development
By recognizing the importance of fairness in AI systems, you can now focus on ensuring responsible AI development, which hinges on addressing biases in data to create fair and ethical machine learning models.
To achieve this, it's vital to prioritize transparency, diversity, and adherence to ethical guidelines. This will help mitigate the impact of biased data on AI ethics.
Collaboration among technologists, ethicists, policymakers, and the community is critical in responsible AI development. By working together, you can detect and correct bias in data analytics, ensuring AI systems align with ethical principles and societal values.
Building fair and responsible AI systems fosters public trust in the ethical use of artificial intelligence technologies. By doing so, you'll be contributing to a future where AI is used for the greater good.
Conclusion
As you gaze into the crystal ball of AI's future, remember that biased data is a ticking time bomb, waiting to release a maelstrom of unfairness and discrimination.
The alarm bells are ringing loud and clear – it's time to take responsibility and guarantee that AI systems are built on a foundation of fairness, transparency, and accountability.
The clock is ticking; will you be the one to set the wheels of change in motion?