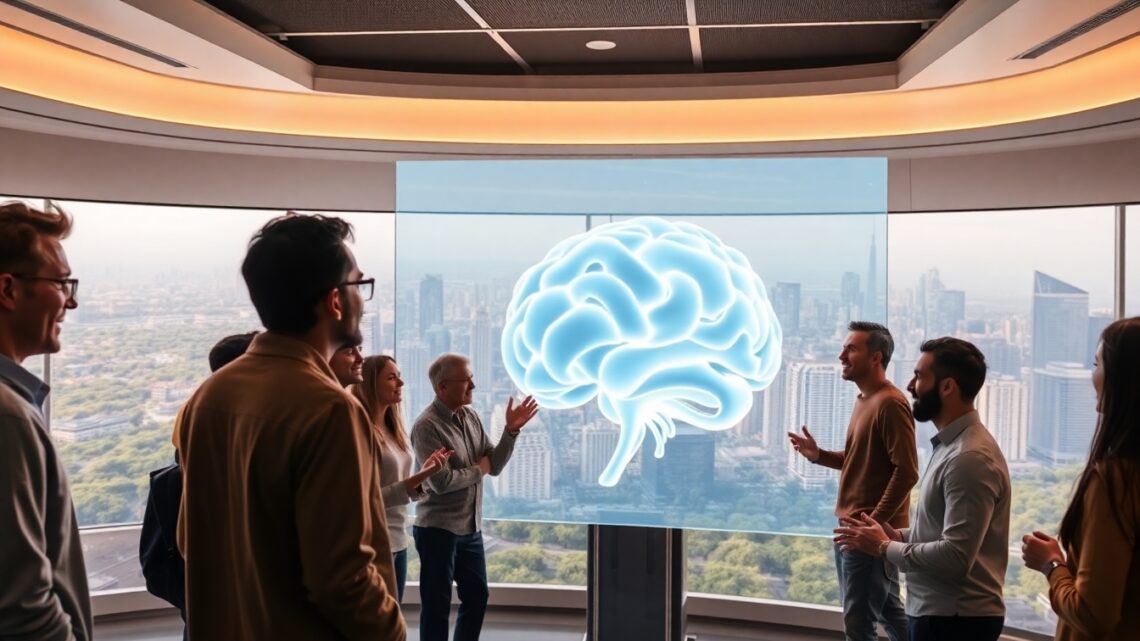
Enhancing AI Evaluation Methods to Match Rising Intelligence Trends
January 4, 2025As artificial intelligence continues to evolve at an unprecedented pace, the importance of refining AI evaluation methods takes center stage. Just as AI models become more sophisticated, so too must our methods of assessing their safety and efficacy. This article delves into the intricacies of AI evaluations, highlighting why advanced evaluation strategies are crucial in today’s rapidly advancing technological landscape.
The Need for Advanced AI Evaluation
With AI systems penetrating diverse sectors, from healthcare to finance, their potential to significantly influence human lives grows alongside. However, this power comes with inherent risks. **Ensuring the safety and reliability of AI models** is essential, not only to protect users but also to cultivate trust in AI technologies.
Challenges in Current Evaluation Methods
Today’s AI evaluation methods are largely inherited from past paradigms and are often inadequate to tackle the complexities of modern AI. These challenges include:
- Over-reliance on Historic Data: Many AI systems are still evaluated using datasets that do not reflect current real-world scenarios.
- Inadequate Testing Environments: Conventional testing settings often fail to simulate the complexity of real-world applications.
- Singular Focus: Current evaluations often prioritize accuracy over other crucial aspects like ethical considerations and robustness.
Innovative Strategies for AI Safety Evaluations
To improve the way AI models are assessed, it is imperative that we introduce new strategies that can cope with the dynamic nature of AI technologies. Here are some proposed methods:
1. Use of Synthetic Data
Synthetic data generation can enhance the evaluation process by providing **diverse and extensive datasets** tailored to specific testing needs. This method helps in understanding how AI models might perform in scenarios that are underrepresented in real-world data.
2. Continuous Evaluation Process
Instead of a one-time evaluation, AI systems should undergo continuous monitoring and assessment. This approach allows evaluators to identify anomalies and adjust the systems in real-time, ensuring they adapt to new datasets and more complex situations.
3. Cross-Domain Testing
By deploying AI models across various unrelated domains, evaluators can discern **unexpected interactions and hidden biases**. This practice provides a broader understanding of an AI’s behavior across different contexts, highlighting aspects that might be overlooked in isolated tests.
Collaboration: A Pathway to Enhanced Safety
Collaboration among AI developers, researchers, and policymakers is crucial to establish unified standards that equate to **comprehensive and seamless evaluation processes**. Creating collaborative platforms that facilitate knowledge sharing can lead to innovative solutions for safety evaluation challenges.
The Path Forward
The rapid advancement of AI intelligence trends necessitates that our evaluation processes not only keep pace but also proactively anticipate future developments. Investing in cutting-edge evaluation technologies and methodologies promises a safer, more reliable future for AI applications. Stakeholders must prioritize these advancements to ensure that AI can reach its full potential, impacting society positively while mitigating risks.
For further reading on the intricacies of AI evaluation methods and their importance in technology today, see the original article on Time.
“`