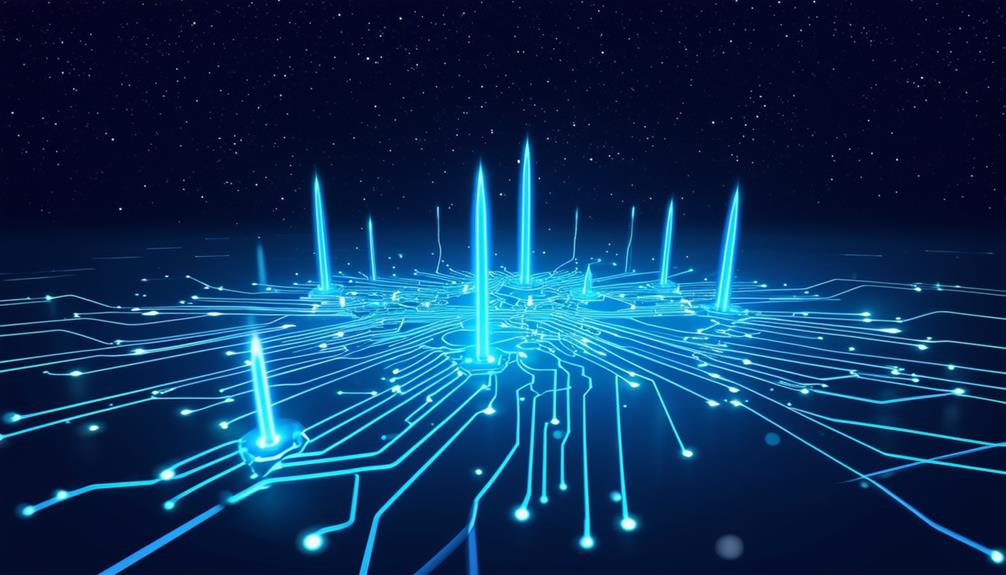
Ensuring Ethical Accountability in AI Systems: 10 Tips
June 11, 2024You're building AI systems that will impact millions, and it's vital to guarantee ethical accountability to prevent unintended harm. Start by implementing bias mitigation strategies, promoting transparency and explainability, and prioritizing privacy and security measures. Establish mechanisms for accountability, incorporate human oversight, and ensure responsible data management practices. Advocate for regulatory frameworks, uphold social responsibility, and conduct regular ethical system audits. By following these 10 tips, you'll be well on your way to building trustworthy AI systems. Now, as you continue to shape the future of AI, discover how these tips can help you create a more ethical and responsible AI ecosystem.
Implementing Bias Mitigation Strategies
To ensure AI systems serve everyone fairly, you must identify and address biases in AI algorithms by training models on diverse and representative datasets to secure impartiality. This is essential because biased AI systems can lead to discriminatory behavior, which can have severe consequences.
To prevent this, you should implement bias detection mechanisms to identify and correct biases in AI systems. This can be achieved by regularly monitoring for biases and adjusting systems to eliminate unfair treatment.
Promoting Transparency and Explainability
As you work to promote transparency and explainability in AI systems, you'll want to focus on three key areas.
You'll need to develop model interpretability methods that allow users to understand how AI decisions are made.
Model Interpretability Methods
By leveraging model interpretability methods, you can uncover the decision-making processes behind AI models, gaining valuable insights into how they arrive at their predictions. This is important in promoting transparency and explainability in AI systems.
Model interpretability methods, such as LIME and SHAP, help you understand how AI models make decisions by highlighting important features. These methods promote transparency and explainability in AI systems by providing insights into the factors influencing model predictions.
Here are some ways model interpretability methods can enhance your understanding of AI systems:
- Uncover biases: Identify biases in your AI models and take corrective action to promote fairness and ethical decision-making.
- Improve accuracy: Analyze feature importance to refine your models and enhance their predictive capabilities.
- Build trust: Enhance transparency and accountability in your AI systems, fostering trust among stakeholders and users.
Data Lineage Tracking
You can guarantee accountability in AI systems by maintaining a detailed record of data lineage, which allows you to trace the origin and journey of data throughout the system. This process, known as data lineage tracking, promotes transparency and explainability in AI decision-making.
By documenting the data's journey, you can understand how it's used, transformed, and processed by AI algorithms. This, in turn, enables you to validate the accuracy and reliability of AI outcomes. Data lineage tracking is essential for compliance with regulations, identifying errors, and addressing biases in AI systems.
It also helps you build trust with stakeholders by demonstrating the ethical handling and utilization of data in AI processes. By implementing data lineage tracking, you can be certain that your AI systems are transparent, reliable, and fair. This is especially important in high-stakes applications, where biased or inaccurate decisions can have serious consequences.
Clear Algorithmic Decisions
When making AI-driven decisions, ensuring clarity around the algorithmic process is vital. Transparency is key to building trust with stakeholders and avoiding potential legal issues. You can't just rely on complex algorithms to make decisions; you need to be able to explain and justify those decisions.
This is where Explainable AI (XAI) comes in – it's essential for promoting transparency and accountability in AI systems.
To achieve clear algorithmic decisions, you should:
Implement XAI techniques** to provide an understandable rationale for AI decisions
Establish transparent algorithmic processes** that are easy to follow and understand
- Regularly review and audit your AI systems to guarantee they're making fair and unbiased decisions
Prioritizing Privacy and Security Measures
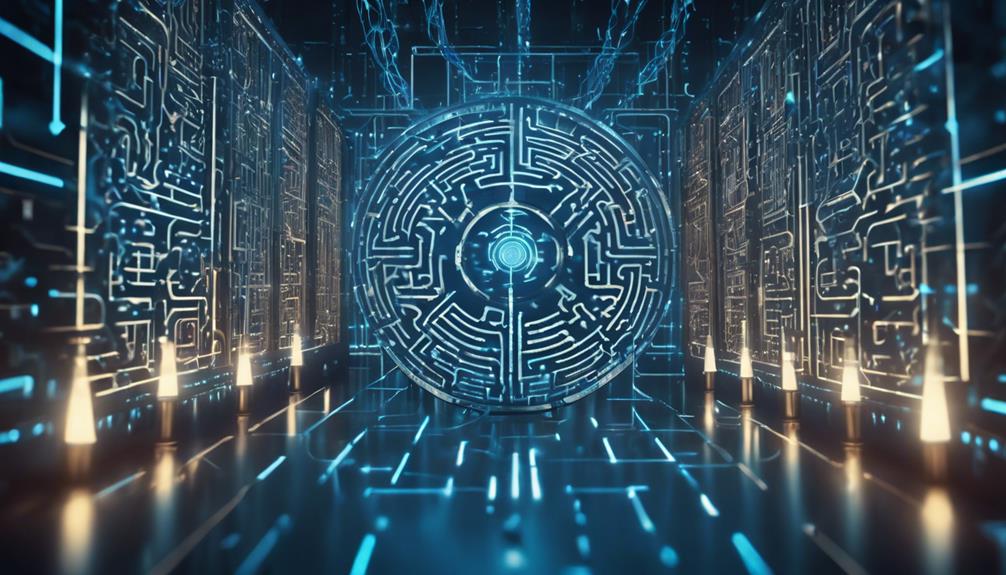
As you design AI systems, you must prioritize privacy and security measures to protect sensitive data.
You'll need to secure data storage and protect user identity to prevent breaches and unauthorized access.
Secure Data Storage
Implementing robust security measures, such as encryption and access controls, is vital for guaranteeing the secure storage of sensitive data in AI systems. As you develop and deploy AI systems, it's important to prioritize secure data storage to protect individual privacy and maintain data integrity.
To achieve this, you can:
- Implement encryption methods, such as homomorphic encryption, to safeguard data both in transit and at rest
- Establish access controls, including multi-factor authentication and role-based access, to restrict unauthorized access
- Develop and enforce data governance frameworks to ensure responsible and ethical data collection, processing, and storage
Protect User Identity
You must prioritize robust security measures to safeguard user identities in AI systems, ensuring that sensitive information remains confidential and protected from cyber threats. This is vital in maintaining user trust and preventing data breaches.
To achieve this, implement encryption and access controls to safeguard user data. Prioritize data protection and secure processing to guarantee user privacy in AI applications. This will help defend against cyberattacks and protect user identities.
Adhere to privacy regulations and standards to maintain the confidentiality of user information. Establish protocols for secure data handling and prevent unauthorized access to user identities. This includes implementing strict access controls, secure authentication, and authorization mechanisms.
By doing so, you'll ensure that user data is protected from unauthorized access, modification, or deletion. Remember, protecting user identities is paramount in building trust in AI systems.
Establishing Mechanisms for Accountability
To establish accountability in AI systems, clear protocols must be put in place to address mistakes or harm caused by these systems. This includes assigning responsibilities to individuals or teams, ensuring that someone is held accountable for AI errors or failures.
Establishing oversight mechanisms and protocols for addressing issues in AI decision-making processes enhances accountability.
You can do this by:
- Regularly monitoring AI systems to detect potential errors or biases
- Implementing feedback loops to correct issues and improve performance
- Ensuring transparency in AI operations and decision-making processes, making it easier to identify and address problems
Incorporating Human Oversight and Review
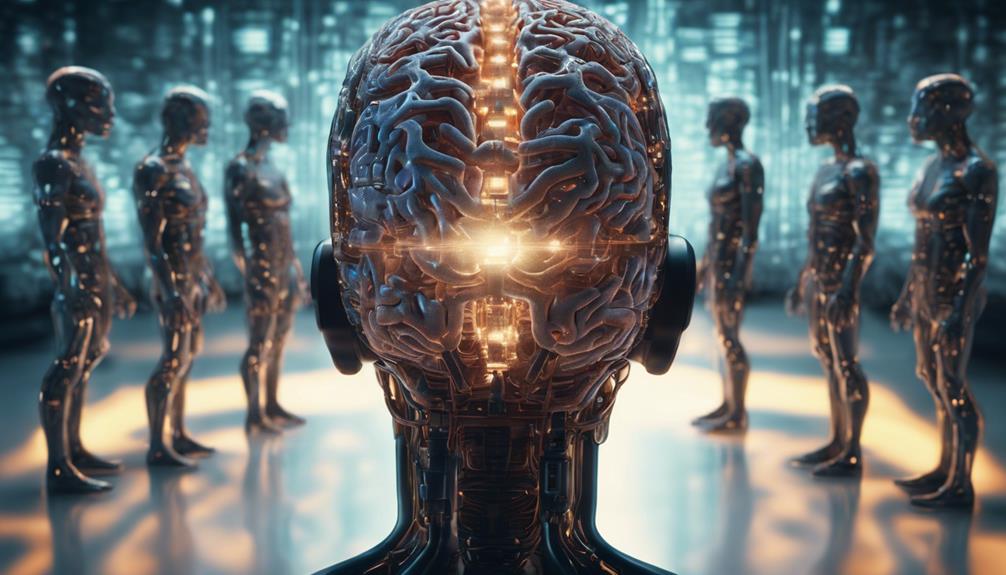
By incorporating human oversight into AI systems, developers can guarantee that critical decisions are carefully reviewed and aligned with ethical standards and organizational values. This oversight is essential in ensuring that AI systems don't operate autonomously, making decisions that may have unintended ethical implications.
You can monitor AI actions and intervene when necessary to prevent errors or bias. Human oversight helps maintain accountability in AI systems by ensuring that decisions align with ethical standards and organizational values. By having humans review AI decisions, you can double-check for accuracy and ethical implications, correcting any mistakes or biases that may arise.
Your feedback and intervention are crucial for improving AI systems over time and addressing any issues that may arise during operation. By incorporating human oversight, you can ensure that AI systems are transparent, explainable, and accountable. This oversight is crucial in building trust in AI systems and ensuring that they operate within ethical boundaries.
Designing for Fairness and Accessibility
As AI systems increasingly interact with diverse populations, designing for fairness and accessibility becomes essential to prevent inherent biases and promote equal opportunities for all individuals.
When you design AI systems, prioritize fairness as a core principle to guarantee equitable treatment for everyone. This means addressing biases that can lead to unfair outcomes and implementing fairness evaluation metrics to prevent discrimination.
To promote inclusivity, incorporate accessibility features into AI interfaces to cater to individuals of all abilities. Consider the interactions people with disabilities may have with AI, making the technology usable for everyone.
You can:
- Design interfaces that are compatible with assistive technologies
- Provide alternative text for visual content
- Guarantee audio descriptions are available for multimedia content
Ensuring Responsible Data Management Practices
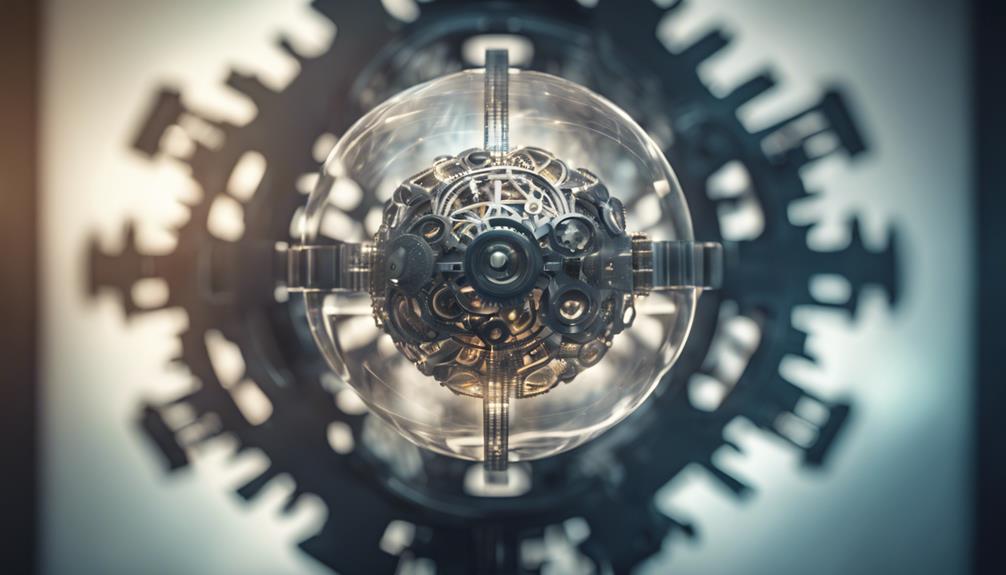
It's essential to guarantee that your AI system's data management practices are responsible and ethical, which involves proper collection, processing, and storage to maintain data integrity and security. You must make sure that your data management practices are ethical to prevent privacy breaches and misuse of sensitive information. Implementing data governance frameworks will help regulate and oversee the handling of data in your AI systems, maintaining accountability.
Ethical data collection is vital to ensure that your AI systems are trained on diverse and representative datasets, minimizing biases and discrimination. When you collect data ethically, you're more likely to develop AI systems that are fair and unbiased.
Proper data management practices in AI development contribute to building trust with users and stakeholders regarding data handling and processing. By ensuring responsible data management, you can ensure that your AI systems are reliable and trustworthy. Remember, it's your responsibility to make sure that your AI systems are designed with ethical data management practices to maintain public trust and prevent potential misuse.
Advocating for Regulatory Frameworks and Governance
You must advocate for robust regulatory frameworks and governance structures to guarantee that AI systems are developed and deployed responsibly, promoting trust and preventing misuse. This involves collaborating with policymakers to shape regulatory frameworks that promote responsible AI use, such as the GDPR and Ethical AI Guidelines.
Establishing governance structures within organizations ensures accountability and compliance with AI ethics, and industry standards like ISO 27001 and IEEE P7000 provide guidelines for ethical AI practices and governance.
To guarantee accountability, you should:
- Establish transparent AI governance frameworks that enhance trust with stakeholders and demonstrate commitment to ethical AI practices
- Implement compliance measures to verify adherence to regulatory frameworks and industry standards
- Foster a culture of ethics and accountability within organizations, promoting responsible AI development and deployment
Upholding Social Responsibility and Ethics
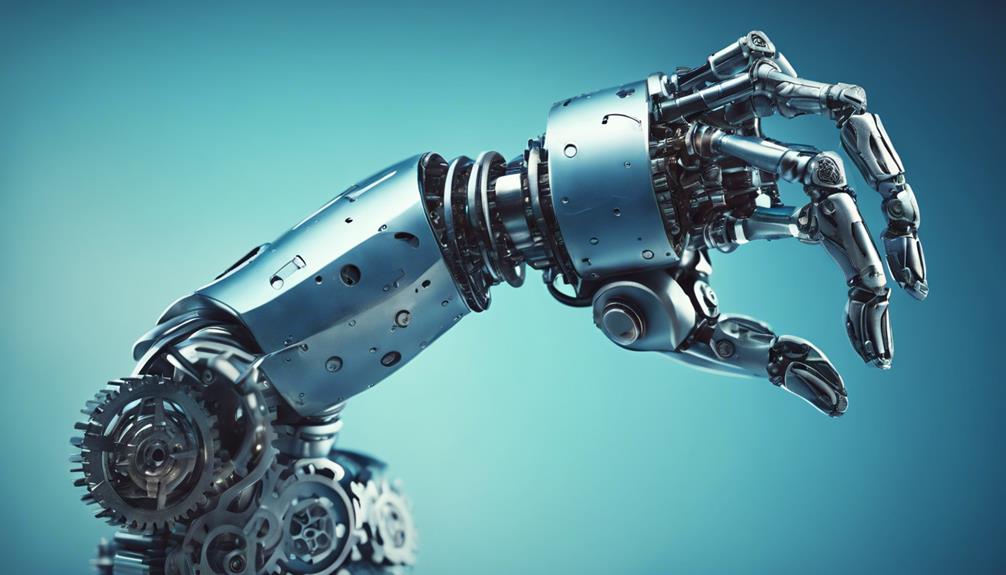
Developers must prioritize ethical practices and take into account the broader societal implications of AI systems to uphold social responsibility and guarantee technology benefits society while minimizing harm. By doing so, you'll make sure that your AI systems are designed with ethical accountability in mind, addressing concerns like bias, discrimination, and privacy.
It's essential to balance innovation with ethical considerations, promoting fairness, transparency, and accountability for positive societal outcomes. As you develop AI systems, remember that social responsibility is key to fostering trust and credibility. By incorporating ethical principles into your design, you'll make certain that your technology benefits society while minimizing harm.
It's your responsibility to take into account the societal impact of your AI systems, prioritizing ethical practices to guarantee a positive effect on the community. By doing so, you'll contribute to a future where AI systems are developed with ethical accountability, promoting fairness and social responsibility.
Conducting Regular Ethical System Audits
As you implement regular ethical system audits, you'll need to determine how often to conduct them to guarantee your AI systems are operating fairly.
You'll also want to develop strategies for detecting systemic biases, which can be subtle but have significant consequences.
Audit Frequency Guidelines
Regular ethical system audits, conducted at a minimum of once a year, are essential for guaranteeing AI systems operate in compliance with ethical standards. This frequency helps identify potential biases, discrimination, or ethical issues within the system, maintaining transparency and accountability.
However, you may want to contemplate conducting audits more frequently, such as semi-annually or quarterly, to enhance accountability and transparency. This is particularly important if your AI system is critical to business operations or has a significant impact on stakeholders.
To guarantee ongoing monitoring of AI systems for ethical performance, establish a clear audit schedule and process. This will help you stay on top of ethical issues and biases, ensuring your AI system operates with integrity.
Some benefits of regular ethical system audits include:
- Ensuring compliance with ethical standards and regulations
- Identifying and addressing biases and discrimination
- Enhancing transparency and accountability in AI decision-making
Systemic Bias Detection
By scrutinizing your AI system's data sources, training processes, and decision outputs, you can uncover hidden biases that might be perpetuating discriminatory behaviors. This is important for maintaining fairness and accountability in AI systems.
Conducting regular ethical audits is essential for systemic bias detection, guaranteeing that your AI system doesn't perpetuate discriminatory behavior. During these audits, you'll examine data sources, training processes, and decision outputs to identify potential biases. By doing so, you'll be able to detect and address systemic bias, enhancing the ethical integrity of your AI technology.
Bias rectification is a critical step in maintaining accountability, and it's only possible through regular ethical audits. By prioritizing systemic bias detection, you'll ensure your AI system operates fairly and without prejudice.
Conclusion
As you've made it this far, you're likely convinced that ethical accountability in AI systems is essential. Now, it's time to take action.
The fate of AI's impact on humanity hangs in the balance. Will you be a pioneer in shaping a responsible AI future, or will you let the consequences of unchecked AI systems unfold?
The clock is ticking. The world is waiting. What's your next move?