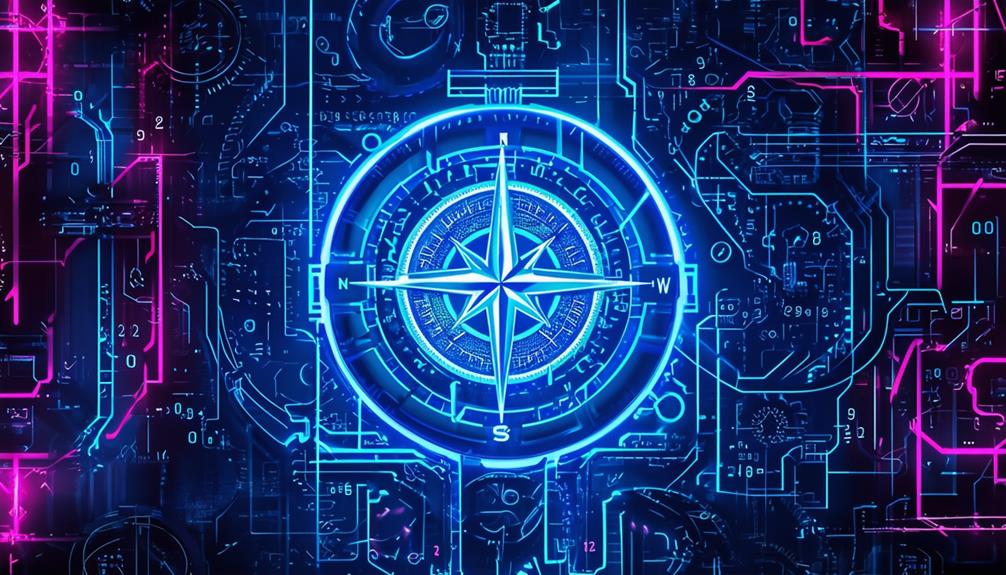
Navigating Ethical Machine Learning Evolution: A How-To Guide
June 8, 2024You're exploring the complex landscape of ethical machine learning evolution to guarantee your organization develops responsible AI systems that prioritize fairness, transparency, and accountability. To get started, foster a culture of experimentation, empowering teams to push boundaries and drive innovation. Embed ethical guardrails early, prioritizing fairness and transparency, and address potential issues proactively. Establish clear governance models, prioritize ethical data practices, and mitigate risks. As you embark on this journey, you'll discover more nuances of responsible AI development and the importance of continuous monitoring and auditing. Ahead, you'll uncover the keys to revealing ethical machine learning evolution.
Fostering a Culture of Experimentation
By embracing a culture of experimentation, you empower your teams to push the boundaries of machine learning innovation, fostering a torrent of creative solutions and breakthroughs. This approach allows teams to test new ideas, driving advancements in machine learning and staying ahead of technological trends. Additionally, it promotes a mindset of continuous improvement, ensuring that your organization remains adaptable to changing ethical guidelines in machine learning.
As you encourage responsible experimentation, you create a safe space for trial and error, enabling rapid learning and adaptation. This, in turn, enables your teams to develop innovative solutions that are both effective and ethical. By embracing experimentation, you demonstrate a commitment to continuous improvement, ensuring that your organization remains at the forefront of machine learning innovation. By doing so, you'll outperform competitors and drive real-world impact.
Embedding Ethical Guardrails Early
As you embed ethical guardrails early in your machine learning development, you'll need to focus on two key areas: ethical design principles and algorithmic accountability checks.
These points are essential in ensuring that your AI solutions are responsible, transparent, and fair from the outset.
Ethical Design Principles
When developing machine learning models, you should prioritize ethical design principles from the outset to minimize unintended consequences and guarantee responsible AI outcomes. This proactive approach secures that your ML models are built on a foundation of fairness, transparency, and explainability, reducing the risk of biases and discrimination. By incorporating ethical AI principles early on, you can address potential issues before they escalate into major problems.
In particular, focusing on fairness and transparency in your ML design helps to prevent biases and promotes inclusive decision-making processes. Additionally, prioritizing transparency and explainability enables you to identify and rectify potential issues more efficiently. By doing so, you can ensure responsible use of your ML models, minimizing harm to individuals and communities.
Algorithmic Accountability Checks
To guarantee responsible AI outcomes, you should integrate algorithmic accountability checks into your machine learning development process from the outset, thereby preventing unintended biases and discriminatory outcomes. By doing so, you'll make sure that ethical considerations that must be addressed are tackled early on. This proactive approach allows you to identify and rectify potential issues before they escalate, upholding ethical standards in your machine learning models.
Algorithmic accountability checks serve as an important safeguard, enabling you to detect biases and unfair outcomes in your algorithms. By embedding ethical guardrails early, you can prevent harm caused by biased AI systems. This is particularly important in high-stakes applications, such as healthcare or finance, where biased decisions can have severe consequences.
Responsible AI Strategies in Practice
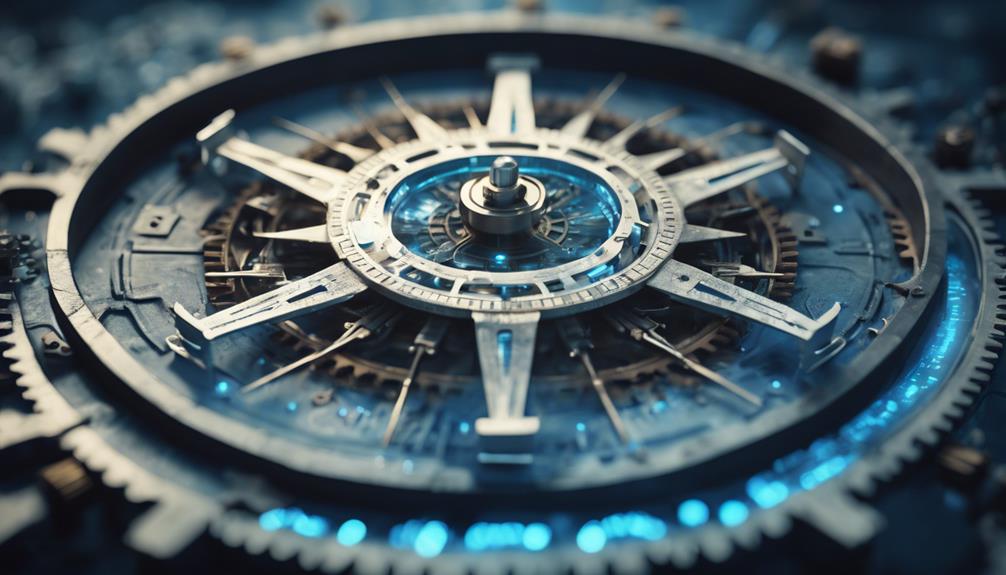
As you explore responsible AI strategies in practice,
you'll need to develop effective AI governance models that guarantee accountability and transparency.
You'll also need to implement ethical data practices that prioritize fairness and privacy.
By focusing on these key areas,
you'll be able to create AI systems that make fair decisions and foster trust.
AI Governance Models
By establishing clear AI governance models, you can guarantee that your organization develops and deploys responsible AI systems that align with ethical principles and values. This is essential in addressing the complex ethical challenges associated with AI technologies.
AI governance models provide a framework for ensuring ethical AI practices and decision-making processes within your organization.
To create an effective AI governance model, consider the following key components:
- Oversight structures: Establish clear lines of authority and decision-making processes.
- Ethical guidelines: Develop guidelines that align with your organization's ethical principles and values.
- Risk management protocols: Identify and mitigate risks associated with AI development and deployment.
- Accountability mechanisms: Ensure transparency and accountability in AI decision-making processes.
Ethical Data Practices
You can guarantee your organization's AI systems are fair, transparent, and accountable by implementing responsible AI strategies that prioritize ethical data practices.
As you develop your machine learning models, it's important to take into account the ethical implications of your data practices. This includes establishing clear ethical guidelines for data collection, storage, and usage to ensure data privacy and security. You must also address potential biases in your data, as these can lead to unfair outcomes and perpetuate societal inequalities.
By prioritizing ethical data practices, you can promote inclusivity and fairness in your AI systems. This involves building ethical frameworks that take into account the societal impact and sustainability of your AI systems. Additionally, inclusive dialogue with stakeholders is critical in ensuring that your AI systems align with ethical values.
Fairness in Decision-Making
Implementing responsible AI strategies in practice involves integrating ethical considerations into the design of AI systems to prioritize fairness and inclusivity in decision-making processes. As you navigate the development of AI systems, prioritizing fairness is crucial to guarantee unbiased outcomes. This can be achieved by implementing ethical guardrails in AI development, which guide the design of AI systems to prioritize fairness and inclusivity.
To promote fairness in decision-making, consider the following key aspects:
- Fairness metrics and evaluation methods: Assess and mitigate biases in AI algorithms to secure fair outcomes.
- Transparency and accountability: Ensure that AI systems are transparent and accountable for their decision-making processes.
- Bias mitigation strategies: Implement strategies to reduce biases in AI algorithms and data.
- Inclusive design: Design AI systems that are inclusive and representative of diverse perspectives.
Prioritizing Transparency and Accountability
As consumers increasingly expect companies to be open about their AI use, prioritizing transparency and accountability in machine learning development has become essential for building trust and credibility.
You're likely aware that transparency in machine learning algorithms is vital for building trust, with studies showing that 73% of consumers are more likely to trust companies that are transparent about their AI use.
Implementing transparency and accountability practices in ML can lead to improved customer satisfaction, as 84% of customers are more likely to trust companies that explain how their AI systems work.
Addressing Algorithmic Bias and Fairness
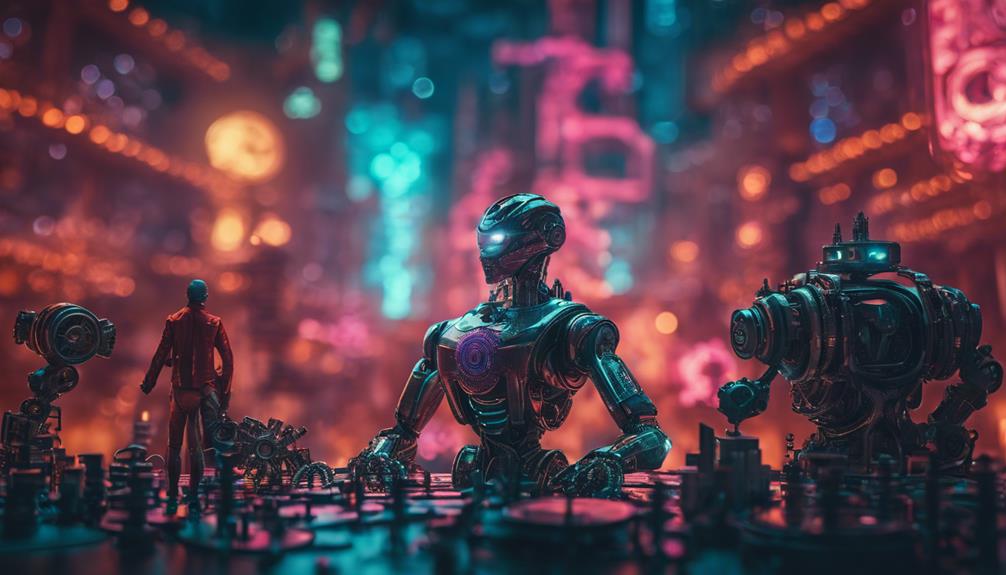
Machine learning models can perpetuate discriminatory biases, leading to unfair outcomes in critical areas like hiring and lending. As you develop and deploy machine learning models, it's crucial to contemplate the ethical implications of bias and fairness.
To mitigate bias and promote fairness, you can employ various techniques, including:
- Data preprocessing: Guarantee your data is diverse and representative to avoid perpetuating existing biases.
- Bias detection: Implement techniques to identify and address bias in your models.
- Model interpretability: Make your models transparent to understand how they're making decisions.
- Fairness metrics: Establish metrics to measure and evaluate fairness in your models.
Ensuring Data Privacy and Security
You've guaranteed fairness and addressed bias in your machine learning models, but now it's time to safeguard the sensitive information used to train them by prioritizing data privacy and security. As you navigate the world of ethical machine learning, it's important to ensure that your data remains confidential and integral throughout the entire process.
To achieve this, implement strong encryption measures to protect your data from unauthorized access. Compliance with data protection regulations like GDPR and CCPA is vital to maintaining privacy and security standards in your ML projects. Regular security audits and updates will help identify vulnerabilities and prevent data breaches in your ML systems.
Prioritizing transparency and accountability in your ML algorithms enhances data privacy and security by promoting trust and ethical practices. By doing so, you'll be ensuring that your machine learning models aren't only fair but also respectful of individuals' rights to data privacy.
Continuous Monitoring and Auditing
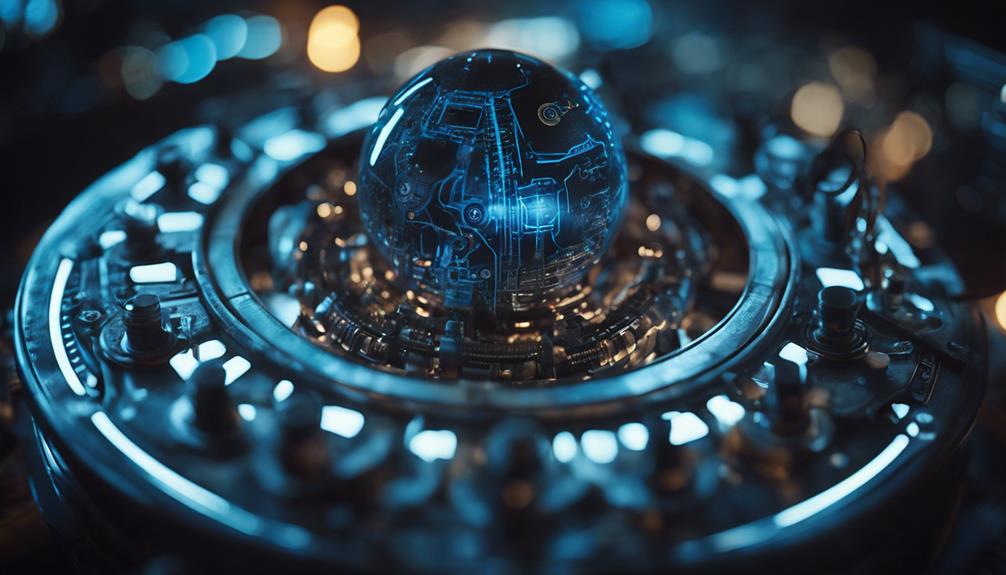
To guarantee the ethical integrity of your machine learning models, ongoing monitoring and auditing are essential for detecting and addressing biases and unfair outcomes in real-time. As you deploy your AI systems, it's vital to implement continuous monitoring and auditing practices to ensure responsible AI deployment.
Here are some essential components to integrate into your monitoring and auditing process:
- Implement bias detection algorithms to identify and address biases in your models
- Utilize model explainability techniques to maintain transparency in your AI decision-making processes
- Conduct regular audits to identify potential ethical issues and ensure compliance with regulations and ethical guidelines
- Establish a system for real-time monitoring to detect and address biases as they arise
Empowering Teams With Education and Training
By investing in thorough education and training programs, organizations can equip their teams with the skills and knowledge necessary to develop and deploy AI systems that uphold ethical standards and promote responsible innovation. You'll want to focus on governance, privacy, and responsible data use to empower your teams in advancing AI ideas.
This education and training will guarantee your teams understand the importance of ethical standards, leading to responsible AI utilization and development.
Becoming prompt engineers is essential for implementing ethical AI practices effectively. By doing so, you'll be able to develop AI systems that prioritize fairness, transparency, and accountability. Additionally, internal applications serve as ideal testing grounds for teams to experiment with AI in an ethical manner.
This hands-on approach will allow your teams to refine their skills and develop a deeper understanding of AI's potential and limitations. By investing in your team's education and training, you'll be well on your way to tapping into AI's potential and promoting responsible AI utilization within your organization.
Conclusion
As you navigate the complexities of machine learning evolution, remember that ethics isn't a destination, but a continuous journey. You've laid the groundwork for responsible AI development, but the real work is just beginning.
Will you prioritize people over profit, or will the consequences of unchecked innovation haunt you?
The future of AI is in your hands – use it wisely.